# Copyright 2022 Google LLC.
#
# Licensed under the Apache License, Version 2.0 (the "License");
# you may not use this file except in compliance with the License.
# You may obtain a copy of the License at
#
# https://www.apache.org/licenses/LICENSE-2.0
#
# Unless required by applicable law or agreed to in writing, software
# distributed under the License is distributed on an "AS IS" BASIS,
# WITHOUT WARRANTIES OR CONDITIONS OF ANY KIND, either express or implied.
# See the License for the specific language governing permissions and
# limitations under the License.
jax.pmap
example using JAXopt.
The purpose of this example is to illustrate how JAXopt solvers can be easily
used for distributed training thanks to jax.pmap
. In this case, we begin by
implementing data parallel training of a multi-class logistic regression model
on synthetic data. General aspects to pay attention to include:
How to use
jax.lax
reduction operators such asjax.lax.pmean
orjax.lax.psum
in JAXopt solvers by using customvalue_and_grad
functions.How
jax.pmap
can be used to transform the solver’supdate
method to easily write custom data-parallel training loops.
To obtain the best performance on Google Colab we recommend:
In
Change runtime type
under the menuRuntime
, selectTPU
for theHardware accelerator
option.Connect to the runtime and run all cells.
NOTE: this example can be easily adapted to support TPU pod slices (e.g. --accelerator_type v3-32
) as well as hosts with one or more GPUs attached.
#@markdown The number of optimization steps to perform:
MAXITER = 100 #@param{type:"integer"}
#@markdown The number of samples in the (synthetic) dataset:
NUM_SAMPLES = 50000 #@param{type:"integer"}
#@markdown The number of features in the (synthetic) dataset:
NUM_FEATURES = 784 #@param{type:"integer"}
#@markdown The number of classes in the (synthetic) dataset:
NUM_CLASSES = 10 #@param{type:"integer"}
#@markdown The stepsize for the optimizer (set to 0.0 to use line search):
STEPSIZE = 0.0 #@param{type:"number"}
#@markdown The line search approach (either `'zoom'` or `backtracking`), ignored if `STEPSIZE > 0.0`:
LINESEARCH = 'zoom' #@param{type:"string"}
Imports and TPU setup
%%capture
%pip install jaxopt flax
import functools
import time
from typing import Any, Callable, Tuple, Union
from absl import app
from absl import flags
# activate TPUs if available
try:
import jax.tools.colab_tpu
jax.tools.colab_tpu.setup_tpu()
except KeyError:
print("TPU not found, continuing without it.")
from flax import jax_utils
from flax.training import common_utils
import jax
import jax.numpy as jnp
import jax.tools.colab_tpu
import jaxopt
import matplotlib.pyplot as plt
import numpy as np
from sklearn import datasets
jax.tools.colab_tpu.setup_tpu()
jax.local_devices()
[TpuDevice(id=0, process_index=0, coords=(0,0,0), core_on_chip=0),
TpuDevice(id=1, process_index=0, coords=(0,0,0), core_on_chip=1),
TpuDevice(id=2, process_index=0, coords=(1,0,0), core_on_chip=0),
TpuDevice(id=3, process_index=0, coords=(1,0,0), core_on_chip=1),
TpuDevice(id=4, process_index=0, coords=(0,1,0), core_on_chip=0),
TpuDevice(id=5, process_index=0, coords=(0,1,0), core_on_chip=1),
TpuDevice(id=6, process_index=0, coords=(1,1,0), core_on_chip=0),
TpuDevice(id=7, process_index=0, coords=(1,1,0), core_on_chip=1)]
Type aliases
Array = Union[np.ndarray, jax.Array]
Auxiliary functions
A minimal working example of how all-reduce mean/sum OPs can be introduced into JAXopt solver’s update
method by overriding jax.value_and_grad
. Note that more complex wrappers are of course possible.
def pmean(fun: Callable[..., Any], axis_name: str = 'b') -> Callable[..., Any]:
"""Applies `jax.lax.pmean` across `axis_name` for all of `fun`'s outputs."""
maybe_pmean = lambda t: jax.lax.pmean(t, axis_name) if t is not None else t
@functools.wraps(fun)
def wrapper(*args, **kwargs):
return jax.tree_map(maybe_pmean, fun(*args, **kwargs))
return wrapper
A small utility to shard Array
s across the available devices:
def shard_array(array: Array) -> jax.Array:
"""Shards `array` along its leading dimension."""
return jax.device_put_sharded(
shards=list(common_utils.shard(array)),
devices=jax.devices())
Custom-loop
The following code uses data-parallelism in the train loop. Through the use_pmap
keyword argument we can deactivate this parallelism. We’ll use this feature later to benchmark the impact of parallelism.
def fit(
data: Tuple[Array, Array],
init_params: Array,
stepsize: float = 0.0,
linesearch: str = 'zoom',
use_pmap: bool = False,
) -> Tuple[np.ndarray, np.ndarray, float]:
"""Fits a multi-class logistic regression model for demonstration purposes.
Args:
data: A tuple `(X, y)` with the training covariates and categorical labels,
respectively.
init_params: The initial value of the model's weights.
stepsize: The stepsize to use for the solver. If set to `0`, linesearch will
be used instead.
linesearch: The linesearch algorithm to use. If `stepsize > 0`, linsearch
will be disabled.
use_pmap: Whether to distribute the computation across replicas or use only
the first device available.
Returns:
The per-step errors and runtimes, as well as the JIT-compile time for the
solver's `update` function.
"""
# Value and grad of the objective function for the solver.
value_and_grad_fun = jax.value_and_grad(jaxopt.objective.multiclass_logreg)
# When `jax.pmap`ing the computation, use JAXopt's option to provide a custom
# `value_and_grad` function to include the desired reduction operators. For
# example, here we decide to average across replicas.
if use_pmap:
value_and_grad_fun = pmean(value_and_grad_fun)
# To override `jax.value_and_grad` in a JAXopt solver, set the flag
# `value_and_grad` to `True` and pass the custom implementation of the
# `value_and_grad` function as `fun`.
solver = jaxopt.LBFGS(fun=value_and_grad_fun,
value_and_grad=True,
stepsize=stepsize,
linesearch=linesearch)
# Apply the `jax.pmap` transform to the function to be computed in a
# distributed manner (the solver's `update` method in this case). Otherwise,
# we JIT compile it.
if use_pmap:
update = jax.pmap(solver.update, axis_name='b')
else:
update = jax.jit(solver.update)
# Initialize solver state.
state = solver.init_state(init_params, data=data)
params = init_params
# If using `pmap` for data-parallel training, model parameters are typically
# replicated across devices.
if use_pmap:
params, state = jax_utils.replicate((params, state))
# Finally, since in this demo we are *not* using mini-batches, it pays off to
# transfer data to the device beforehand. Otherwise, host-to-device transfers
# occur in each update. This is true regardless of whether we use distributed
# or single-device computation.
if use_pmap: # Shards data and moves it to device,
data = jax.tree_map(shard_array, data)
else: # Just move data to device.
data = jax.tree_map(jax.device_put, data)
# Pre-compiles update, preventing it from affecting step times.
tic = time.time()
_ = update(params, state, data)
compile_time = time.time() - tic
outer_tic = time.time()
step_times = np.zeros(MAXITER)
errors = np.zeros(MAXITER)
for it in range(MAXITER):
tic = time.time()
params, state = update(params, state, data)
jax.tree_map(lambda t: t.block_until_ready(), (params, state))
step_times[it] = time.time() - tic
errors[it] = (jax_utils.unreplicate(state.error).item()
if use_pmap else state.error.item())
print(
f'Total time elapsed with {linesearch} linesearch and pmap = {use_pmap}:',
round(time.time() - outer_tic, 2), 'seconds.')
return errors, step_times, compile_time
Boilerplate
Creates dataset, calls fit
with and without jax.pmap
, makes figures.
def run():
"""Boilerplate to run the demo experiment."""
data = datasets.make_classification(n_samples=NUM_SAMPLES,
n_features=NUM_FEATURES,
n_classes=NUM_CLASSES,
n_informative=50,
random_state=0)
init_params = jnp.zeros([NUM_FEATURES, NUM_CLASSES])
errors, step_times, compile_time = {}, {}, {}
for use_pmap in (True, False):
exp_name: str = f"{'with' if use_pmap else 'without'}_pmap"
_errors, _step_times, _compile_time = fit(data=data,
init_params=init_params,
stepsize=STEPSIZE,
linesearch=LINESEARCH,
use_pmap=use_pmap)
errors[exp_name] = _errors
step_times[exp_name] = _step_times
compile_time[exp_name] = _compile_time
plt.figure(figsize=(10, 6.18))
for use_pmap in (True, False):
exp_name: str = f"{'with' if use_pmap else 'without'}_pmap"
plt.plot(jnp.arange(MAXITER), errors[exp_name], label=exp_name)
plt.xlabel('Iterations', fontsize=16)
plt.ylabel('Gradient error', fontsize=16)
plt.yscale('log')
plt.legend(loc='best', fontsize=16)
plt.title(f'NUM_SAMPLES = {NUM_SAMPLES}, NUM_FEATURES = {NUM_FEATURES}',
fontsize=22)
plt.figure(figsize=(10, 6.18))
for use_pmap in (True, False):
exp_name: str = f"{'with' if use_pmap else 'without'}_pmap"
plt.plot(jnp.arange(MAXITER), step_times[exp_name], label=exp_name)
plt.xlabel('Iterations', fontsize=16)
plt.ylabel('Step time', fontsize=16)
plt.legend(loc='best', fontsize=16)
plt.title(f'NUM_SAMPLES = {NUM_SAMPLES}, NUM_FEATURES = {NUM_FEATURES}',
fontsize=22)
return errors, step_times, compile_time
Main
print("num_samples:", NUM_SAMPLES)
print("num_features:", NUM_FEATURES)
print("num_features:", NUM_CLASSES)
print("maxiter:", MAXITER)
print("stepsize:", STEPSIZE)
print("linesearch (ignored if `stepsize` > 0):", LINESEARCH)
print()
errors, step_times, compile_time = run()
print('Average speed-up (ignoring compile):',
round((step_times['without_pmap'] / step_times['with_pmap']).mean(), 2))
num_samples: 50000
num_features: 784
num_features: 10
maxiter: 100
stepsize: 0.0
linesearch (ignored if `stepsize` > 0): zoom
Total time elapsed with zoom linesearch and pmap = True: 5.88 seconds.
Total time elapsed with zoom linesearch and pmap = False: 20.39 seconds.
Average speed-up (ignoring compile): 7.08
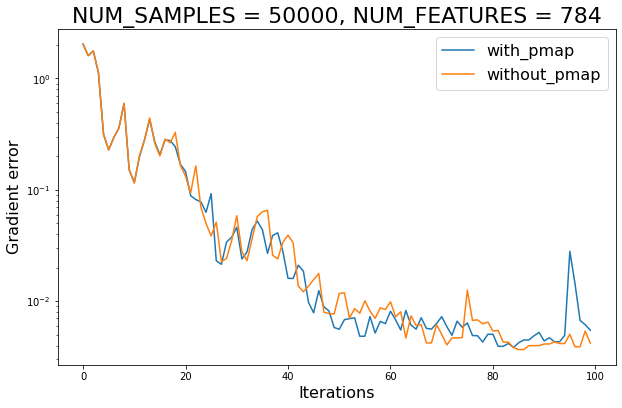
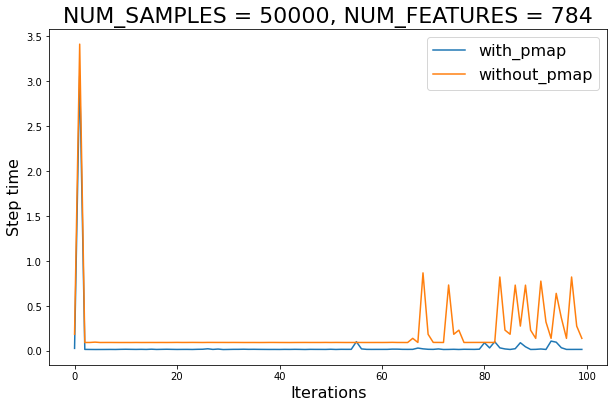